Introduction
Business intelligence and analytics (BIA) is quickly becoming a necessary technology in modern business practices. Conceptually, its implementation and applications not only enhance operational efficiency and product development but also helps contribute towards improving customer relationships (Ajah & Nweke, 2019; Al-Okaily, et al., 2022). In the banking sector particularly, BIA enables experts and managers to make well-informed and timely decisions, which is argued by (Nithya & Kiruthika, 2021) to have a positive impact on productivity, and profitability due to the shorting of.
Today, according to (Bany Mohammad, et al., 2022), BIA has evolved to help in shaping the existing corporate identity, allowing businesses to bridge the gap between strategic use of technology for enhancing business growth and sustainability to the existing and new data. In practice, the emphasis on the use of data to drive informed decision-making not only provides businesses with an advantage in the ever-dynamic market but also highlights the necessity of banks to make investments in research and development (R&D). (Al-Okaily, et al., 2021) in their work on the success of enterprise resource planning (ERP) systems argue that data is a key driver for future business practices through enabling efficient processing and effective support for strategic decisions, which subsequently influences corporate performance.
The continual growth of data led to the emergence of big data and related technologies in both the business and academia fields. With such growth, BIA has become a key tool to organizations’ decision-making processes due to its capability to allow business to process huge amounts of data into actionable insights (Mohammed, et al., 2024). Moreover, recent emergence of disruptive technologies like Internet of Things (IoT), cloud computing, and artificial intelligence has resulted in significant increase in the number of financial transactions conducted through different media (Kravchenko, 2023). Such media include internet banking, mobile banking, etcetera through which more data is being generated making it essential for businesses to adopt new approaches to obtain reliable information for which to assess customer needs for developing and/or improving individual products (Kravchenko, 2023). Ideally, the cause effect of this is the need of businesses to have methods for high-quality collection of data, processing, structuring, and analysis which when combined become big data and big data analytics (Kravchenko, 2023).
Objective
Evolution of data, big data and associated technologies, and in particular analysis and computing has been an important factor in the growth and sustainability of the business and finance sectors. Generally, the actual role of big data and analytics as a tool for supporting decision making can vary from informing decisions on improving processes to decisions on business expansion and product development. To this end, the current work seeks to conduct a systematic literature review research on the role of big data and business analytics in the banking and financial management sector.
Method
Search Strategy
Table 1 below provides an overview on the information extraction process adopted to collect studies that were reviewed.
Table 1: Search Strategy for Obtaining academic studies for the study
Characteristic | Value |
Period of interest | Studies published after 2015 |
Number of Search Terms | 6 |
Search Terms | (“big data” OR “large-scale data” OR “data analytics” OR “data mining” OR “data science”) AND (“business analytics” OR “business intelligence” OR “predictive analytics” OR “prescriptive analytics” OR “descriptive analytics” OR “data-driven decision-making”) AND (“competitive advantage” OR “strategic advantage” OR “organizational performance” OR “market competitiveness” OR “sustainable competitive advantage”) AND (“decision-making” OR “strategic decision-making” OR “managerial decision-making” OR “decision support systems”) AND (“banking” AND “financial management” AND “financial services” AND “financial institutions”) AND (“2016” OR “2017” OR “2018” OR “2019” OR “2020” OR “2021” OR “2022” OR “2023” OR “2024”) AND (“google scholar”) |
Number of studies reviewed | Original extracted Studies: 20, Reported/Filtered Studies: 6 |
Inclusion Criteria | Academic Research studies that were published after the year 2021 that examined aspects of big data and supporting decision making and/or competitive advantage and other related terms (“synonyms” of decision making, competitive, and advantage ). |
Literature Synthesis
This section organizes previous studies by themes and extracts information related to the current work’s objective.
Big Data Analytics (BDAs) and Performance
In literature, it is defined as the process of creating architectures and new technologies to extract economic value from a large amount of data. This involves capturing data at high velocity, discovery and/ or analysis. Over the years, BDAs has garnered significant attention from policymakers, given its role in decision making (Zhu & Yang, 2021). According to (Mikalef, et al., 2018), many companies have intensified their BDAs initiatives to gain crucial insights and maintain a competitive edge with both business practitioners and academics viewing BDAs as a promising frontier for fostering innovation, competition, and productivity in the banking sector.
(Gul & Al-Faryan, 2023) conducted an empirical analysis to examine the influence of data-driven decision-making (DDDM) on productivity within Pakistan’s banking sector, considering the sector’s data analytics capability. The variables obtained from the primary survey used to collect data are explained in table 2 below.
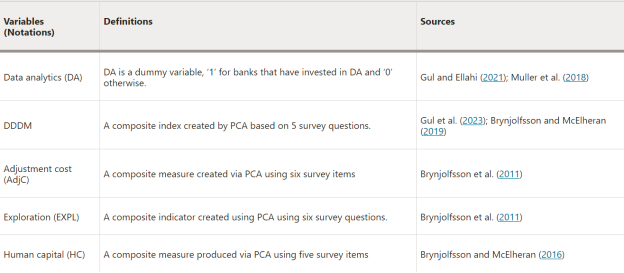
Output (Y) in the study by (Gul & Al-Faryan, 2023) is the dependent variable that is measured following the function on Cobb-Douglas production because output in the baking industry is relatively different from that of other industries. As such, the study used loans and deposits as the output variable in place of units sold. Table 3 below shows the construction of the survey variables
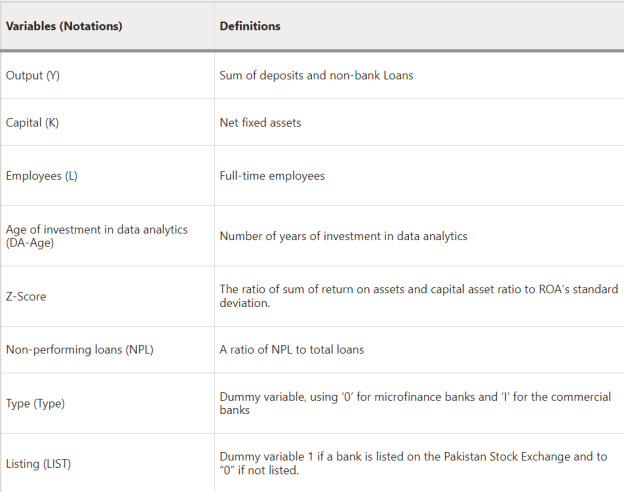
Table 4 shows the descriptive statistics for different variables.
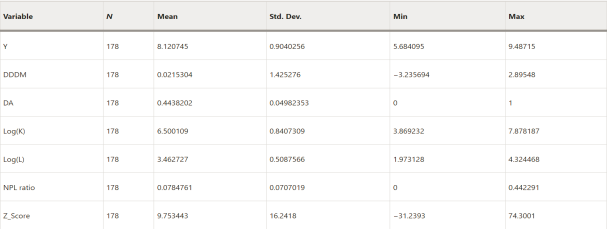
From table 1 above, the average use of data analytics was approximately 0.44 indicating that on average slightly less than half of the banks invested in data analytics.
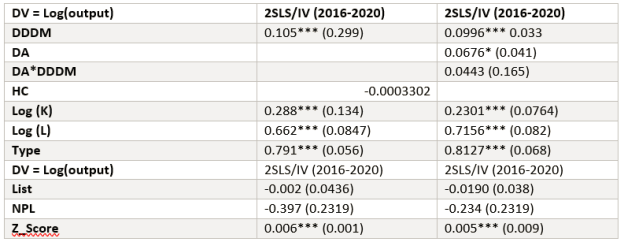
After controlling for non-performing loans (NPL), Z_Score, non-performing loans, type of banks, as well as a bank’s listing on the Stock exchange (List), (Gul & Al-Faryan, 2023) showed that the use of data-driven decision making in the banking sector leads to an increase in the productivity of banks by between 9% and 10% and that the investment of banks in data analytics promoted business productivity.
Big Data and Predictive Analysis
Utilizing insights from Big Data not only yields financial benefits by reducing costs or enhancing productivity but also unlocks new business prospects (Al-Lozi, et al., 2022). These opportunities are rooted in decisions reliant on Big Data analysis, which typically lead to more effective outcomes. Through Big Data Analytics, historical data undergoes processing using advanced tools to evaluate extensive volumes of structured, unstructured, and semi-structured data collected from different sources (Asad, et al., 2020).
Timely decision making based on Big Data is a crucial factor for achieving a competitive advantage in the business and service sectors. Many businesses globally regard competitive advantage as the primary benefit of employing data analysis for predictive purposes. Banking businesses, in particular, stand out for leveraging predictive analysis through Big Data analytics for areas such as credit cards, credit history assessment, financing methods, and investment opportunities (Patil, et al., 2018). Big data frameworks like Hadoop have been utilized alongside machine learning techniques for addressing banking sector issues like detecting fraud with about 72% accuracy when using decision trees and logistic regression models (Patil, et al., 2018). Table 6 below shows a summary of the utilization of big data in finance by (Patil, et al., 2018).
Table 6: Usage of Big Data in Financial Sector: Source: The role of big data in financial sector A review paper
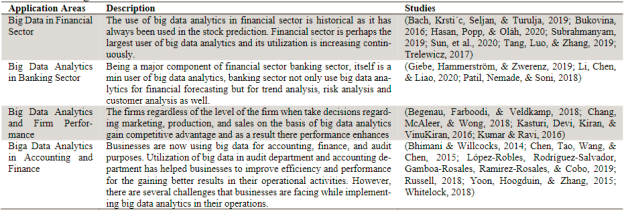
(Patil, et al., 2018) as observed above posit that big data analytics is a primary driver for decision-making across different business sectors including marketing, production, and sales which brings about competitive advantage in the banking industry regardless of the level of application.
Discussion and Conclusion
The primary goal of this work was to conduct a systematic review to examine the role of big data and data analytics in the banking sector in providing competitive edge. Overall, our findings show that there is huge potential of big data analytics and data-driven decision-making to act as decision support tools through the use of technologies like machine learning and data mining. Arguably, better decisions improve organizational performance and therefore allows businesses to gain competitive advantage.
BDAs, according to existing literature are defined by their ability to facilitate the extraction of economic value from large volumes of data among businesses. Through these insights, banks can learn among other business aspects, the current market trend, customer behavior, and competition trends. The findings further show that investment in data analytics has a significant effect on business output in the industry. This supports the argument that it is essential for banks to invest in better data analytics technology to enhance productivity. These arguments are supported by the empirical analyses, such as the study by Gul & Al-Faryan (2023) which show that there is a positive impact of DDDM and DA on productivity within the banking sector.
Following the findings from the current research, it can be argued that current business shifts from traditional decision-making to data-driven decision making plays a key role in enabling banks to improve their decision-making process and gain competitive edge through reducing costs associated with processes like marketing, risk management, and resource optimization. That is, big data analytics allows businesses to extract useful insights from the ever-increasing data generated from growing sources.
Limitations
The current work adopts a literature review approach. Use of findings from literature review poses the risk of transferring bias from the original research and since the primary data was not verified, the validity of the current work depends on the validity of the primary studies. Therefore, the findings from the current work cannot be generalized to a larger population until further analysis is conducted.
References
Ajah, I. & Nweke, H., 2019. Big data and business analytics: Trends, platforms, success factors and applications. Big Data Cogn. Comput., 2(32).
Al-Lozi, E., Alfityani, A., Alsmadi, A. & Al-hazimeh, A., 2022. The role of big data in financial sector: A review paper. International Journal of Data and Network Science, Volume 6.
Al-Okaily, A., Al-Okaily, M. & Teoh, A., 2021. Evaluating ERP systems success: Evidence from Jordanian firms in the age of the digital business.. VINE J. Inf. Knowl. Manag. Syst, Volume ahead-of-print.
Al-Okaily, A., Al-Okaily, M., Teoh, A. & Al-Debei, M., 2022. An Empirical Study on Data Warehouse Systems Effectiveness: The Case of Jordanian Banks in the Business Intelligence Era. EuroMed J. Bus., Volume ahead-of-print.
Asad, M., Altaf, N., Israr, A. & Khan, G., 2020. Data analytics and SME performance: A bibliometric analysis.. s.l., Research Gate.
Bany Mohammad, A., Al-Okaily, M., Al-Majali, M. & Masa’deh, R., 2022. Business Intelligence and Analytics (BIA) Usage in the Banking Industry Sector: An Application of the TOE Framework. J. Open Innov. Technol. Mark. Complex, 4(8), p. 189.
Gul, R. & Al-Faryan, M. A. S., 2023. From insights to impact: leveraging data analytics for data-driven decision-making and productivity in banking sector. Humanities and Social Sciences Communications, 10(2023), p. 660.
Kravchenko, I., 2023. The role and use of big data in banking and financial services, s.l.: DICEUS.
Mikalef, P., Pappas, I. & Krogstie, J., 2018. Big data analytics capabilities: a systematic literature review and research agenda. Inf Syst E-Bus Manage, 16(2018), pp. 547-578.
Mohammed, A. B., Al-Okaily, M., Qasim, D. & Al-Majali, M. K., 2024. Towards an understanding of business intelligence and analytics usage: Evidence from the banking industry. International Journal of Information Management Data Insights, 4(1).
Nithya, N. & Kiruthika, R., 2021. Impact of Business Intelligence Adoption on performance of banks: A conceptual framework. J. Ambient. Intell. Humaniz. Comput., Issue 2021, pp. 3139-3150.
Patil, S., Nemade, V. & Soni, P. K., 2018. Predictive modelling for credit card fraud detection using data analytic. Procedia Computer Science, 132(2018), pp. 385-395.
Zhu, X. & Yang, Y., 2021. Big Data Analytics for Improving Financial Performance and Sustainability. Journal of Systems Science and Information, 9(2), pp. 175-191.